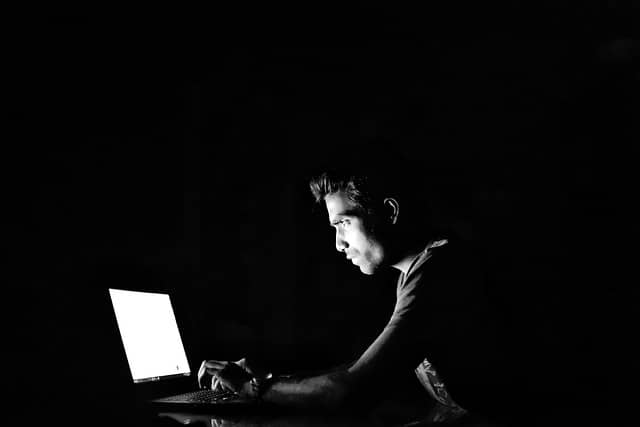
Introduction
The presence of noise in web data can be good and bad, depending on the context and the analysis goals. In some cases, noise in web data can provide valuable information and insights that may not be apparent in clean, preprocessed data. For example, in social media analysis, noise such as typos can reveal important trends and sentiments among users that would otherwise be missed if the data was clean. Similarly, in web traffic analysis, bots and spam traffic can provide insights into the sources and types of traffic to a website. On the other hand, in some cases, noise in web data can obscure or distort the underlying patterns and insights the business is trying to uncover. For example, in web search analysis, irrelevant or spam results can make it difficult to identify users’ true intent and interests. In web analytics, bot traffic or other fraudulent traffic can distort the metrics used to measure website performance in web analytics. Whether noise in web data is good or bad depends on the specific context and goals of the analysis. In general, it’s important to carefully consider the sources and types of noise in web data and determine how to handle them appropriately based on the desired outcomes of the analysis.
Key characteristics of meaningful noisy web data
While noisy web data can distort underlying patterns and insights that businesses are trying to uncover, it can provide insight that may not be apparent in clean, processed data. Here are some key characteristics of meaningful noisy web data:
- Diversity: Meaningful noisy web data from different sources provide diverse perspectives and viewpoints. This can help to uncover hidden insights and trends that may not be apparent in more homogeneous data.
- Volume: Good noisy web data should be sufficiently large in volume to ensure enough information to draw meaningful conclusions. Large datasets can also help to mitigate the effects of individual outliers or noise points.
- Relevance: Some noisy web data can be relevant to a problem statement or a research question. Irrelevant noise can make drawing meaningful insights from the data more difficult.
- Contextual information: meaningful noisy web data should come with contextual information, such as metadata or user profiles, that can help understand the noise’s sources and motivations.
- Consistency: While noise by definition is not consistent, good noisy web data should exhibit some degree of consistency in terms of the types of noise present and how it is distributed across the dataset. This can help to identify patterns and trends in the noise that may be useful for analysis.
Examples of good noisy web data
- Social media data: Social media platforms are rich noisy data sources that can provide valuable insights into user behaviour and sentiment. This data can include text, images, videos, user profiles, and metadata. Noisy features of social media data might include slang, typos, emojis, and hashtags that users employ to express themselves.
- Search engine data: Search engine data can include search queries, clicks, and web page content, and can be used to analyse user intent and preferences. Noisy features of search engine data might include spelling errors, ambiguous queries, irrelevant search results, and click fraud.
- Web traffic data: Web traffic data can provide insights into the volume, sources, and patterns of traffic to a website. Noisy features of web traffic data might include bot traffic, spam traffic, and click fraud.
- User-generated content: User-generated content such as reviews, ratings, and comments can be valuable sources of noisy data that can provide insights into user preferences and opinions. Noisy features of user-generated content might include bias, exaggeration, and spam.
- Online marketplace data: Online marketplaces such as eBay or Amazon can provide a rich source of noisy data on product prices, reviews, and ratings. This data can be used to analyse pricing trends, consumer behavior, and product quality. Noisy features of online marketplace data might include fraud, fake reviews, and spam.
Type of noise in web data
There are several types of noise that can be present in web data. Some of the most common types of noise in web data include:
- Sampling noise: This type of noise occurs when the sample of data collected from the web does not represent the entire population. This can happen for reasons such as non-random sampling or selection bias.
- Measurement noise: This type of noise occurs when the measurements or observations of the data are affected by errors or inaccuracies. For example, measurement noise might include errors due to sensor inaccuracies or rounding errors.
- Random noise: This type of noise is inherent to the data and cannot be explained or predicted. For example, random noise might include errors in web data due to unpredictable fluctuations or environmental changes.
- Systematic noise: This type of noise is also inherent to the data but can be explained or predicted. For example, systematic noise might include errors in web data due to consistent biases in the measurement system or sampling process.
- Outlier noise: This type of noise occurs when individual data points are significantly different from the rest of the data, often due to errors or anomalies. Outlier noise can make identifying patterns or trends in the data difficult.
- Semantic noise: This type of noise occurs when the meaning or interpretation of the data is ambiguous or unclear. For example, semantic noise might include the use of slang or idioms in social media data that is difficult to interpret.
In summary, noisy web data can be leveraged to uncover insights that may not be apparent from cleaner, preprocessed data. However, it is important to carefully consider the sources and types of noise in the data and take steps to handle them appropriately based on the desired outcomes of the analysis.