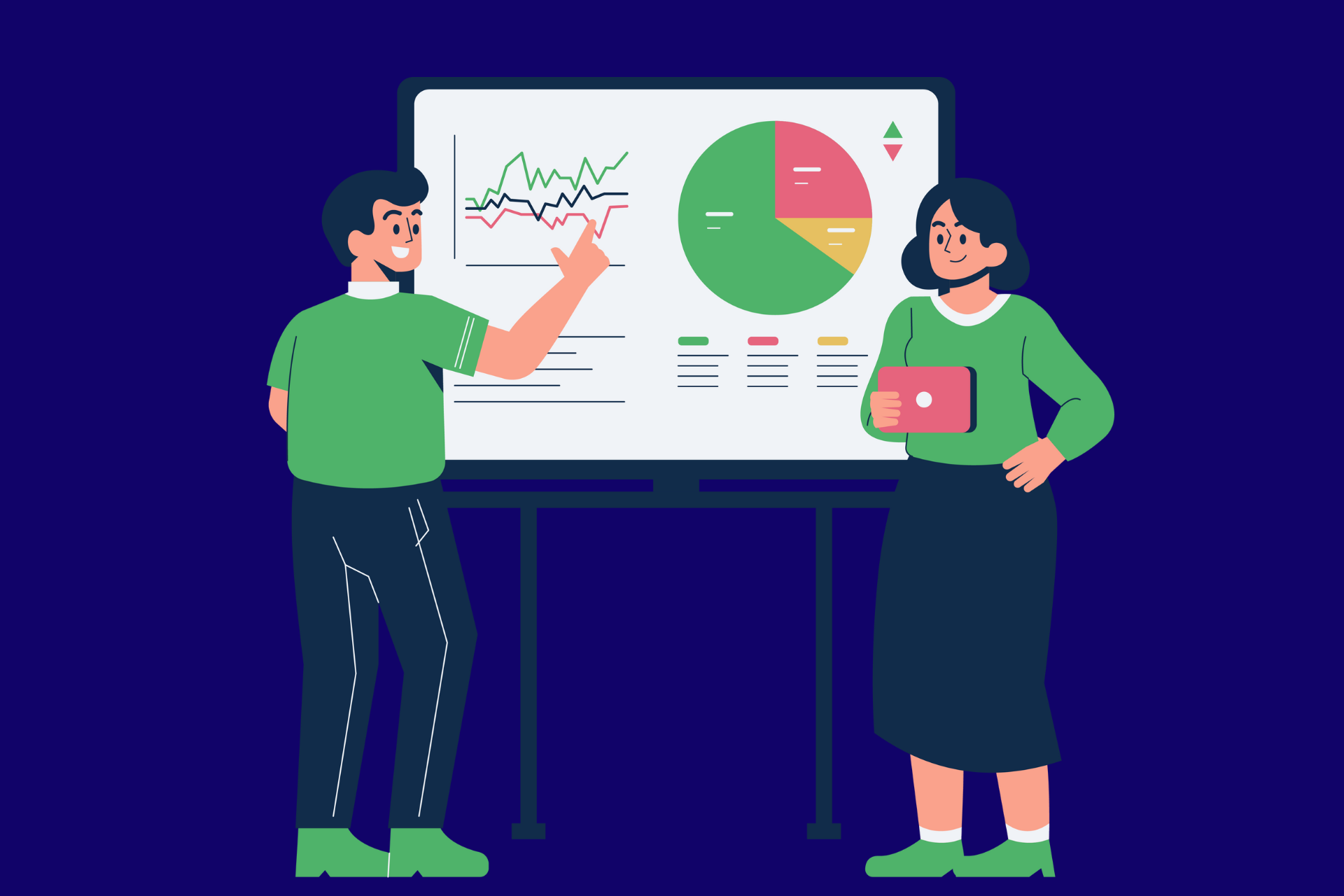
Introduction
Augmented analytics is a data analytics approach that uses machine learning and natural language processing to automate the analytics processes typically done by a specialist or a data scientist. Augmented analytics relieves the dependence on data analysts or scientists by automating insights generation within a business using advanced machine learning and AI algorithms. As its name suggests, augmented analytics enhances the efforts of humans by taking on repetitive data gathering and prep tasks, freeing time for data scientists to focus on more strategic and creative tasks, such as asking better business questions, finding innovative data sources, and interpreting insights strategically.
The beauty of augmented analytics lies in its ability to take data from many channels, like Google Ads, Facebook, Shopify, or any other platform, and apply powerful machine learning algorithms to discover critical insights that save money and increase your bottom line. As a new wave in BI technology, it builds upon and improves upon older data analytics models, making insights available to all business users, including those with limited skills or knowledge.
Benefits of using Augmented analytics
Organisations can benefit from using augmented analytics because it helps them quickly derive meaningful insights from multiple data sources, and they do not need any data science technical skills or expertise. In embedded analytics software, augmented analytics adds greater efficiencies to the data analytics process, equipping business users with tools that answer their questions within seconds and helping businesses get a 360-degree view of their corporate data. In addition, it enables massive time savings and cuts down on manual processes, using round-the-clock automated data preparation techniques to predict user needs, and accelerating data analytics through artificial intelligence and machine learning techniques.
Here are some examples of how augmented analytics can provide benefits:
- Improved efficiency: For example, an organisation might use augmented analytics to automate the process of preparing and cleaning data for analysis. This can save time and effort for data analysts, who might otherwise spend hours manually processing and organising data.
- Enhanced accuracy: An organisation might use augmented analytics to identify patterns and trends in data that humans might miss. For example, an AI-powered tool might be able to detect a subtle but significant correlation between two variables that a human analyst might overlook.
- Greater accessibility: Augmented analytics can make data analysis and decision-making more accessible to a wider range of users, including those with limited technical skills or expertise. For example, an organisation might use natural language processing (NLP) to enable non-technical users to ask questions and get insights from data using plain language.
- Increased agility: An organisation might use augmented analytics to make real-time data-driven decisions, enabling it to respond more quickly to changing business conditions. For example, an e-commerce company might use real-time analytics to optimise its inventory based on demand trends and customer behaviour.
- Cost savings: Augmented analytics can help organisations reduce the costs associated with data analysis and decision-making by automating tasks and processes and reducing the need for specialised personnel. For example, an organisation might use automated machine learning (AutoML) to build and optimise machine learning models without hiring additional data scientists.
Examples of how augmented analytics can be used
- Customer segmentation: A company might use augmented analytics to analyse customer data and identify trends and patterns that can be used to segment customers into different groups. This can help the company to tailor its marketing and sales efforts to different customer segments in a more targeted and effective way.
- Predictive maintenance: A manufacturer might use augmented analytics to analyse data from its production equipment to identify patterns that can be used to predict when maintenance is likely to be needed. This can help the company to proactively schedule maintenance and avoid equipment failures that could disrupt production.
- Fraud detection: A financial services company might use augmented analytics to analyse data from its transactions and identify patterns that could indicate fraudulent activity. This can help the company quickly and accurately detect and prevent fraud, saving it significant time and resources.
- Inventory optimisation: A retailer might use augmented analytics to analyse data from its sales and inventory to identify patterns that can be used to optimise its inventory levels. This can help the retailer to reduce excess inventory and improve its profitability.
- Employee performance: A company might use augmented analytics to analyse data from its employees’ work patterns and productivity to identify trends and patterns that can be used to improve performance. This can help the company to identify areas for improvement and take targeted actions to increase productivity.
Conclusion
Because of the augmented analytics machine learning capabilities combined with natural language processing and automated insights, augmented intelligence allows data to be analysed at scale, providing rapid insights to drive time-sensitive decisions. Companies are using augmented analytics to cut time and costs for data preparation, find insights from data, communicate those insights, and act. Both business users and C-suite executives can leverage augmented analytics to extract greater value from data, without requiring special skills or IT know-how.